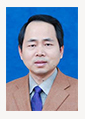
Li Kaicheng
Huazhong University of Science and Technology, China
Title: Power quality disturbances detection based on strong trace filter
Biography
Biography: Li Kaicheng
Abstract
Power quality disturbances (PQDs) detection plays a very important role in smart grid and customer safety, power quality evaluation, and power monitoring. Transient PQDs has strong impact on both grid and customer and bring serious consequences. Therefore, researches on transient PQDs detection are expected urgently in these days. The main challenges of transient PQDs detection are the interference caused by background noise and frequency deviation. This paper proposed a new method to detect transient power quality disturbances (PQDs) based on strong trace filter (STF). By appropriate filter model design, when there are stationary PQDs, the STF works as same as Kalman filter, while when there are transient disturbances, the STF indicates each sudden change of the distorted waveform by the fading factor (FF). The FF can also reveal which parameter of the signal component is changing and its sensitivity to sudden change can be tuned by the soften factor easily to avoid noise influence. Besides, the STF is a simple algorithm, which can be easily implemented on embedded system for real-time and time-varying detection. In addition, STF does not require synchronous sampling. Both simulation and experiment suggest that the STF is a good solution for transient PQDs detection. There is no doubt that the STF has a good prospect on transient PQDs detection.
Recent Publications (minimum 5)
1.Harper C (2009) The neuropathology of alcohol-related braindamage. Alcohol Alcohol 44:136-140.
2. C.-C. Liao, Enhanced RBF network for recognizing noise- ridingpower quality events, IEEE Trans. Instrum. Meas., 59(6):1550–1561, Jun. 2010.
3.M. Zhang, K. Li, and Y. Hu, Classification of power quality disturbances using wavelet packet energy and multiclass support vector machine,COMPEL, Int. J. Comput. Math. Elect. Electron. Eng., 31(2):424–442, 2012.
4. L. Angrisani, P. Daponte, M. D’Apuzzo, and A. Testa, A measurement method based on the wavelet transform for power quality analysis, IEEE Trans. Power Del., 13(14):990–998, Oct. 1998.
5.C.-Y. Lee and Y.-X. Shen, Optimal feature selection for power- quality disturbances classification, IEEE Trans. Power Del., 26(4): 1250–1257, Oct. 2011.